
How to Scale #DataOps, with Romain Dardour (Hull)

This article includes key takeaways from Romain Dardour’s talk on how to scale data ops. Check out the full video on Youtube or get the latest from our Scale series.
5 key best practices
Best practice #1: It’s all about infrastructure
“The data you need to work in one tool is usually in another tool.” Romain quotes himself on this one to explain the mission of any data ops team: setting up an infrastructure that makes data accessible and usable to everyone in the company. An all too common mistake is to focus on quantity, attempting to retain every possible scrap of data. This leads data ops teams to ignore the more pressing problem: more and more teams (growth, marketing, sales, support — to name just a few) require reliable, actionable data. As the company’s internal “data dealer”, data ops teams need to focus on making sure these teams can obtain and use that data. And though it may seem like a given, in most cases customer data is fragmented and unreliable…
Best practice #2: Scale when it hurts
Romain isn’t the first of our guests to share this tip. But, when it comes to data, the question to ask yourself is: “Does my CRM fit in my brain?” Or, in other words: do you have a clear picture of your clients and their health just from memory. If the answer is yes, don’t scale. If the answer is no, get ready to take your data to the next level…
Note: Growing your use of data typically follows through these steps: first, exporting and importing CSVs from one tool to another, with obvious limitations. Second, plugging Zapier between all of your tools, with exponential difficulty as you add new tools. Third, in-house integrations between your tools, which can drain resources from your engineering team. Actually scaling data, however, is something entirely different (see Best practice #3).
Best practice #3: Start small
Data politics can get ugly. But it’s necessary to get everyone aligned at the start of your data scaling process. Step 1: get everyone in a room. Objective: agree on one modest scenario in which data can help. Step 2: figure out what data you need to fulfil the scenario, and where it is located. Objective: accomplish your scenario — your data ops MVP — in a clean, repeatable way. Step 3: unify your data. Objective: ensure that profile fields are consistent from one tool to the next to create an accurate view of all your customer data points. Step 4: create custom logic. Objective: now that you’re working on a reliable data set, you can start creating segments that are consistent and playing with attribution models that fit your business.
Note: As Romain notes, opportunities for increased customization (and competitiveness) expand dramatically when your customer data is unified. A good example of this is Mention’s simple customer lifecycle, from first touch to conversion, which is available here. As the lead moves further down the funnel, previously blind touchpoints (like an anonymous website visit) can be mapped out (through cookies that can allow you to link a blog reader with the email in the request a demo field) and offer a deeper understanding of your customer’s needs and what brought them to you.
Best practice #4: Look for the T-shape
Data ops teams are at the intersection of two realms: marketing and engineering. So, when you hire, be on the lookout for profiles that combine those skills. Either a T-shaped marketer with a soft spot for tech (able to work on SQL, Python, JavaScript) or engineers that have expanded their scope into marketing. Their role isn’t purely based on analysis — unlike, say, Data Scientists — so technical proficiency is a must for Data Engineers who will be in charge of laying out your data infrastructure.
Best practice #5: Clean up after yourself
When it comes to organizing your data ops team, Romain suggests taking a leaf out of Drift’s playbook. Drift have structured a team with dedicated engineers to supply all of the company’s departments with data. The contract they have with all of the other departments is simple: they need to prove that their experiment works. And if it doesn’t: they are responsible for undoing it. By having a dedicated team for data ops, other teams (marketing, growth, sales, customer success) can rely on clean data. And by enforcing a simple “show me it works” rule, the data and stack can remain clean.
Note: Before working your way up to a dedicated Data Ops team, it makes sense to integrate data ops into the growth team because: a) like growth, data ops is a cross-departmental function and b) they require a similar skill set.
2 key mindsets
Mindset #1: Forget about the tools
“What tools should I use?” — the question often comes up when it comes to setting up data processes. For Romain, debating tools is superfluous. What matters is how connected your data is, and how easy it is to iterate on it. Instead of focusing on your data stack, start with your scenarios. The challenge of data ops is not to collect more and more data, but to make that data more accessible, more usable, and more homogenous in all of your tools.
Mindset #2: Get your priorities in order
Your number 1 priority, you guessed it, making iteration on your customer data fast and secure. Romain insists on the difficulty of doing this in the absence of a staging environment. Unlike your product team, your data team carries out actions that have the potential to make or break your sales pipeline. So it’s important to stay cautious and aware of this as you manipulate data. It might break. It might screw up. It might blow up in your face. Be rigorous, keep a cool head, and keep pushing until all your data is unified — that’s the hardest part!
Subscribe to get notified of the latest Scale talks.
About Romain Dardour:
Romain is co-founder of Hull and a mentor at TechStars. His tip for scaling businesses to get the most out of their data: keep it simple. Whether it’s for sales, marketing, operations, getting bogged down too early in vast volumes of data is pitfall #1. Pitfall #2 comes as startups grow, not knowing how to structure their processes and leverage that data. Follow Romain on Twitter.

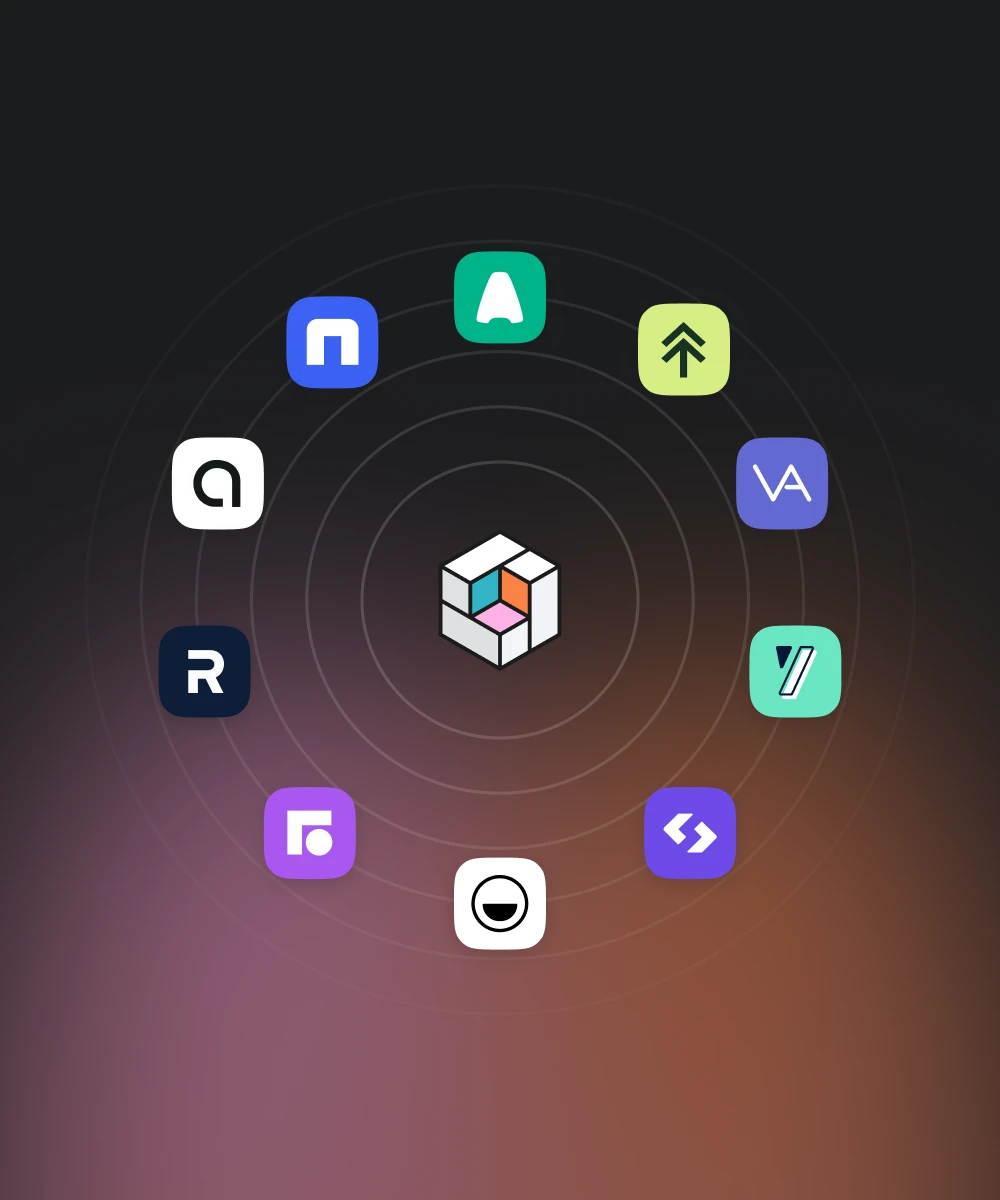